Lukas Vermeer's manual sample ratio mismatch (SRM) checker
Quickly learn if your data is randomized properly or not
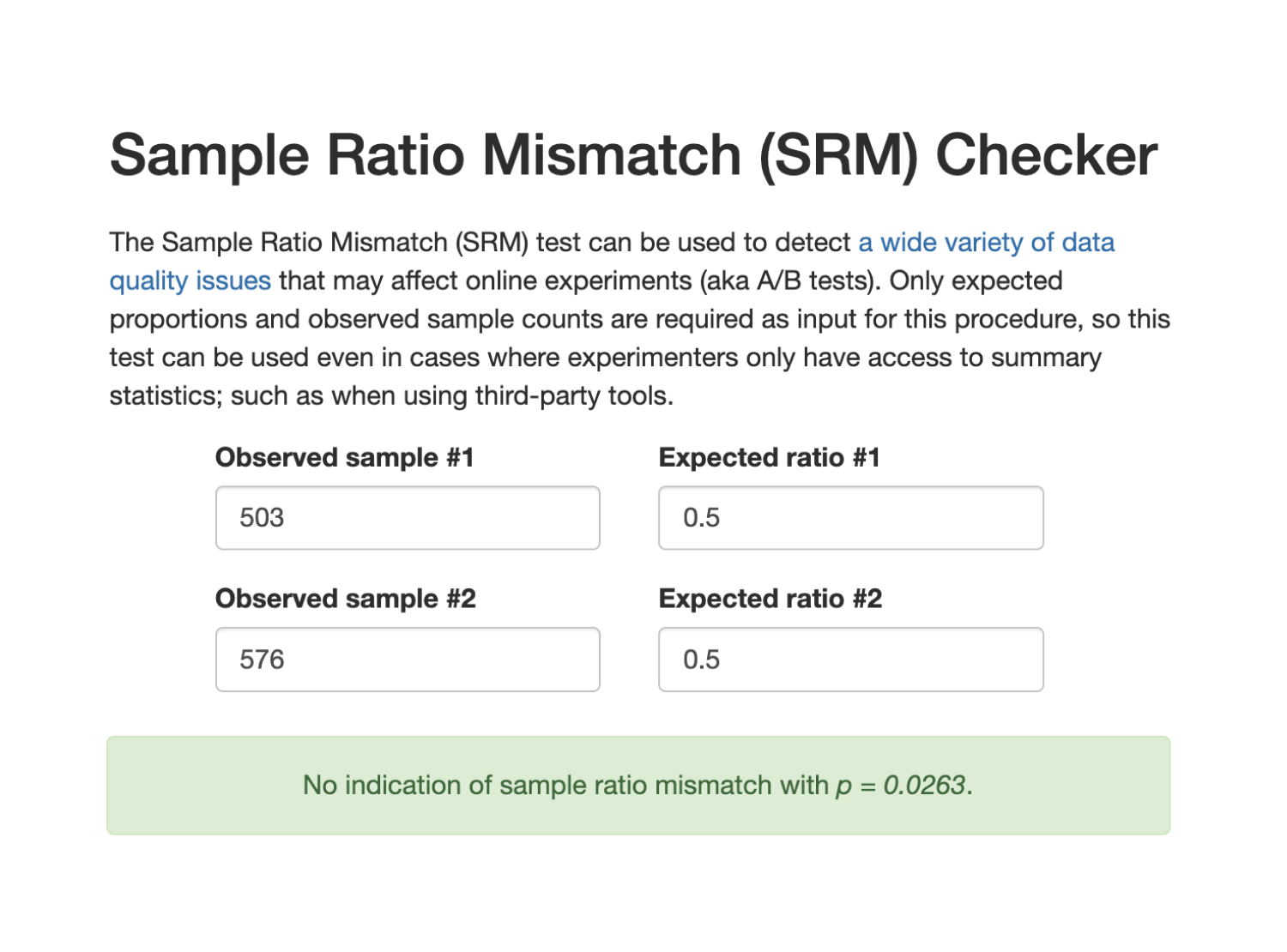
Randomization is the hidden power behind A/B testing. When randomized properly, the confounds in your data are completely removed. This allows you trust any cause/effect relationship you might observe.
To manually check for a sample ratio mismatch (SRM) head over to Lukas Vermeer's sample ratio mismatch checker at LukasVermeer.nl. Here's how to use it:
- Plug in the ratio you wanted your sample split into. By default the checker is pre-populated with .5 (aka 50%) for the "Expected ratio #1" and "Expected ratio 2" form fields. This means the intended split was 50/50. This is the most common split ratio, but it's also possible to choose any other ratio that makes sense in your situation.
- Go to your A/B testing tool and get the sample size for both the control and the treatment groups. You can usually find these numbers by looking for something labeled "visitors," "users," or "participants."
- Plug in the sample size numbers you observe in your A/B testing tool. These numbers go in the fields labeled "Observed sample #1" for your control and "Observed sample #2" for your treatment.
- Look at what the colored banner below the fields says. The checker works on-the-fly, so there's no need to push any buttons or do anything else.
How to read the manual SRM check result
If the banner is green and says, "No indication of sample ratio mismatch with p = ..." then there is no sample ratio mismatch in your data. The figure below illustrates what a result without an SRM looks like.
If the banner is yellow and says, "Warning. Possible sample ratio mismatch detected with p < 0.0001. The observed sample size in treatment does not match the expected proportion of the total sample size. This is an indicator for randomisation failure or missing data issues. Comparative statistics may be invalid as a result."
This simply means you're like to have an SRM in your data, and you should not trust the result.
MOBILE: Lukas Vermeer's manual sample ratio mismatch (SRM) checker
Quickly learn if your data is randomized properly or not
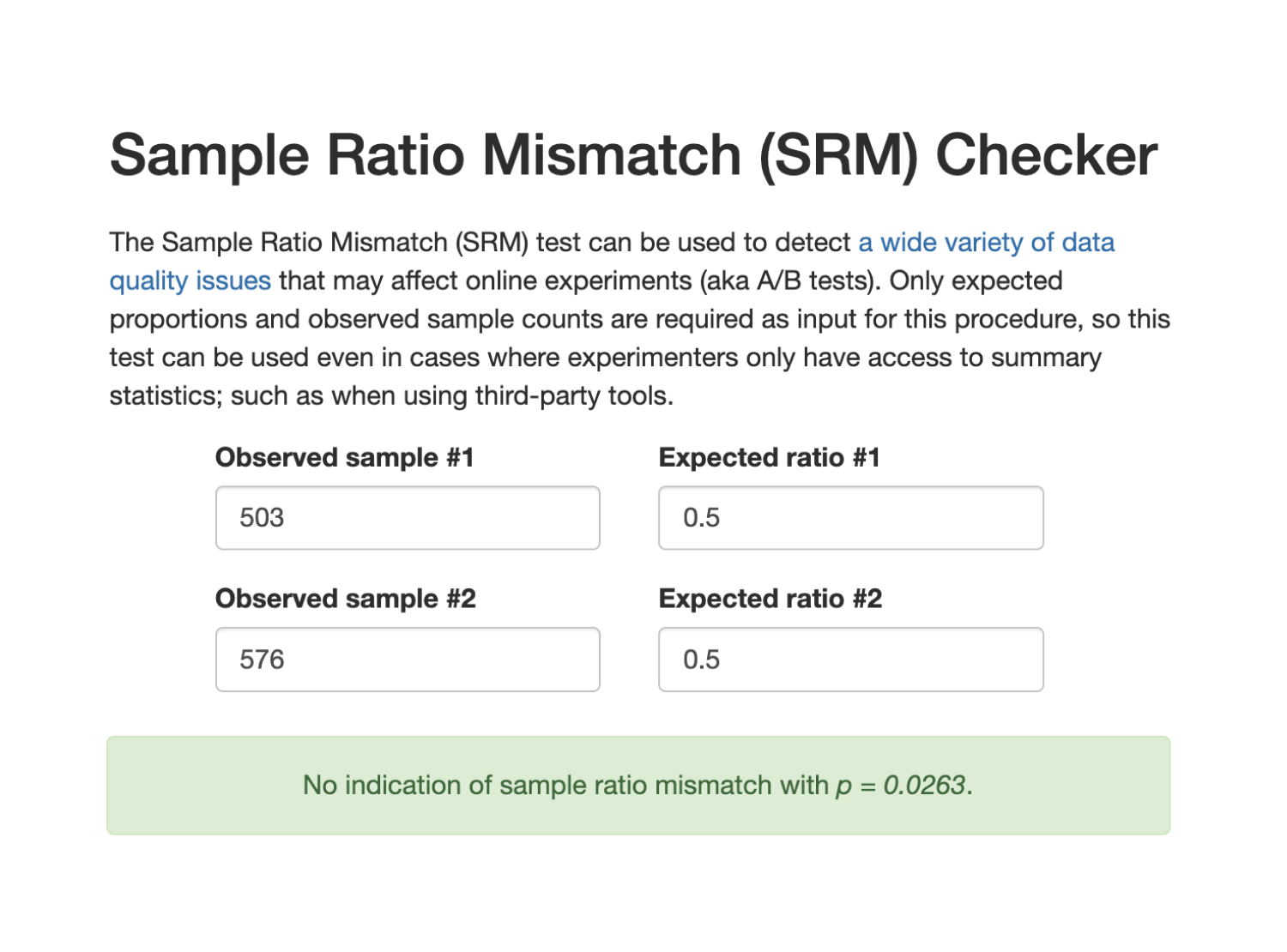
Randomization is the hidden power behind A/B testing. When randomized properly, the confounds in your data are completely removed. This allows you trust any cause/effect relationship you might observe.
To manually check for a sample ratio mismatch (SRM) head over to Lukas Vermeer's sample ratio mismatch checker at LukasVermeer.nl. Here's how to use it:
- Plug in the ratio you wanted your sample split into. By default the checker is pre-populated with .5 (aka 50%) for the "Expected ratio #1" and "Expected ratio 2" form fields. This means the intended split was 50/50. This is the most common split ratio, but it's also possible to choose any other ratio that makes sense in your situation.
- Go to your A/B testing tool and get the sample size for both the control and the treatment groups. You can usually find these numbers by looking for something labeled "visitors," "users," or "participants."
- Plug in the sample size numbers you observe in your A/B testing tool. These numbers go in the fields labeled "Observed sample #1" for your control and "Observed sample #2" for your treatment.
- Look at what the colored banner below the fields says. The checker works on-the-fly, so there's no need to push any buttons or do anything else.
How to read the manual SRM check result
If the banner is green and says, "No indication of sample ratio mismatch with p = ..." then there is no sample ratio mismatch in your data. The figure below illustrates what a result without an SRM looks like.
If the banner is yellow and says, "Warning. Possible sample ratio mismatch detected with p < 0.0001. The observed sample size in treatment does not match the expected proportion of the total sample size. This is an indicator for randomisation failure or missing data issues. Comparative statistics may be invalid as a result."
This simply means you're like to have an SRM in your data, and you should not trust the result.
More useful resources
What you need to know about sample ratio mismatches (SRMs)
Randomization within experimentation is important. It’s how we isolate the change we aim to learn about. When randomization goes wrong, you can get an SRM.
Go to resourceThe Good Experimental Design toolkit
The Good Experimental Design toolkit Templates and checklist to level-up your experimental design The Good Experimental Design Toolkit by Erin Weigel. As Ronald Fisher learned, experiment data is only as […]
Go to resourceA/B testing tool comparison
Speero’s A/B testing tool comparison website Helping you find the right experimentation tool quickly and easily Speero’s A/B testing too comparison website includes a comprehensive list of options. If you’re […]
Go to resourceMore useful resources
What you need to know about sample ratio mismatches (SRMs)
Randomization within experimentation is important. It’s how we isolate the change we aim to learn about. When randomization goes wrong, you can get an SRM.
Go to resourceThe Good Experimental Design toolkit
The Good Experimental Design toolkit Templates and checklist to level-up your experimental design The Good Experimental Design Toolkit by Erin Weigel. As Ronald Fisher learned, experiment data is only as […]
Go to resourceA/B testing tool comparison
Speero’s A/B testing tool comparison website Helping you find the right experimentation tool quickly and easily Speero’s A/B testing too comparison website includes a comprehensive list of options. If you’re […]
Go to resource